Research Interest
Computer Vision
Image and Video Restoration
Underwater Vision
Segmentation of Aerial Images
Image Memorability
Robotic Vision
Zero Shot Learning
Active Learning
Medical Imaging
Human Gait Analysis
Detection and Rehabilitation for Osteoarthritis
Adversarial Perturbation
Adaptive Video Coding
Media Forensics
Steganography and Steganalysis
Watermarking and Data Hiding
Underwater Computer Vision
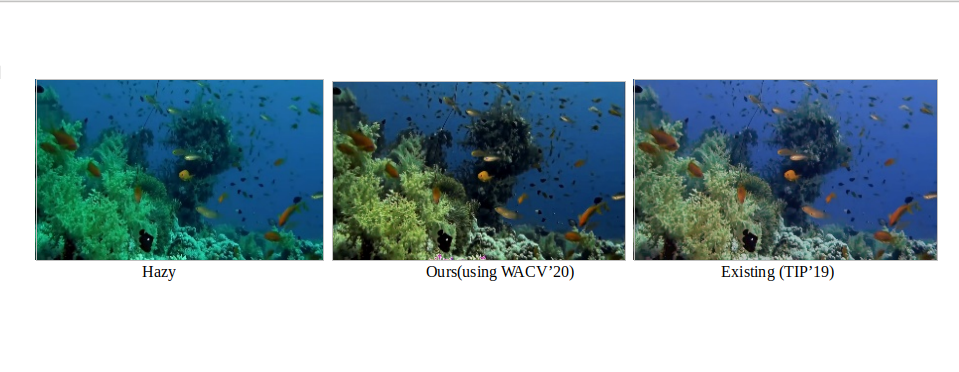
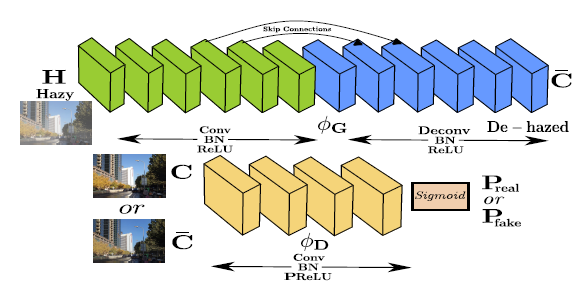
The problem of underwater image enhancement has been given considerable importance in recent times due to its vast application area in marine engineering and aquatic robotics. The two foremost reasons that make the underwater image restoration difficult are scattering and color distortion. Similar to the problem of single image dehazing, underwater image dehazing also consists of two major phases : (1) Removal of haze, followed by the post-processing step, and (2) Color correction. Mathematically, the phenomenon of underwater image dehazing based on the transmission-based haze segmentation can be written as H(x) = C(x)T(x) + L(x)(1 - T(x)), where H, C, T, and L denote Hazy, Clean, Transmission map, and Atmospheric light map, respectively. The deep learning-based method proposed in Sharma et al. (WACV’20) can be used to generate the de-hazed images in the underwater scenario, as shown in the following figure.
[1]. Prasen Kumar Sharma, Priyankar Jain, and Arijit Sur. "Scale-aware Conditional Generative Adversarial Network for Image Dehazing". Winter Conference on Applications of Computer Vision (WACV 2020), USA.